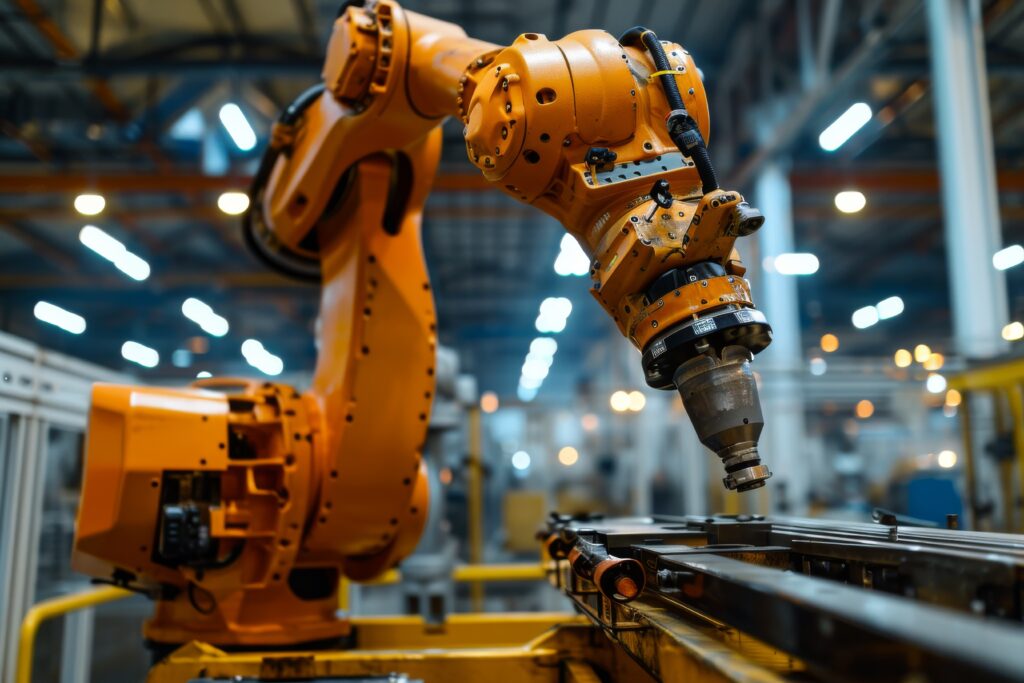
An automatic hydraulic system installed and working in a complex factory operation. (Photo courtesy NineTwoThree)
With an accuracy rate of more than 99 percent, AI-aided predictive maintenance systems can greatly reduce costs and improve uptime for manufacturers.
BOSTON—Maintaining uptime in manufacturing is paramount. To prevent work stoppages, manufacturers have traditionally relied on two strategies: reactive and preventive maintenance.
With reactive maintenance, equipment is fixed when it breaks. This almost always leads to costly downtime, which, in some cases, can reach hundreds-of-thousands of dollars an hour. To reduce these failures, manufacturers also rely on preventive maintenance—regularly replacing parts to avoid unexpected downtime. However, this approach increases maintenance costs because equipment is often replaced earlier and more often than necessary. It also does not account for components that break unexpectedly.
With artificial intelligence (AI) and machine learning (ML), manufacturers are now increasingly turning to predictive maintenance to identify, detect, and address issues in real time. By collecting data and employing advanced analytics, manufacturers can increase the lifespan of machines, ensure equipment is running more efficiently, and reduce waste. In addition, avoiding unexpected component failures ensures that broken parts do not negatively impact other components of the machine or put operators in danger.
Benefits of predictive maintenance for manufacturing
Whether a manufacturer has a high degree of data maturity or is just becoming aware of the benefits of predictive maintenance, employing machine learning is, in many cases, proving to be worth the investment.
Recently, a U.S.-based manufacturer utilizing a complex hydraulic system was looking to reduce the risks associated with unexpected downtime by developing a predictive maintenance system. Its goal was to create an ML model that would predict the degradation of specific components, including the cooler, valve, pump, and hydraulic accumulator.
When a single component malfunction can disrupt an entire hydraulic system, detecting faults before they become larger issues is critical. The data science team working on the hydraulic system had already installed condition monitoring sensors and gathered a dataset with labeled degradation levels for each separate component. However, turning that into a working AI program was beyond their expertise.
The company eventually turned to NineTwoThree, an AI engineering firm based out of Boston, to create a preventive, machine learning-based system. The manufacturer had a goal of reaching 95 percent accuracy.
For this project, the AI engineers deployed new sensors and new settings, and they changed elements of the system. This provided a raw dataset based on many physical sensors’ measured parameters during the load cycle of the hydraulic system. Virtual sensors were also added to the design.
Data analysis
Data collection and classification usually make up a large portion of any machine learning project. Predictive maintenance projects can become extremely complex when dealing with a large amount of data, and too much data can create accuracy issues.
With all the sensors and load cycles, the project’s data originally exceeded a total of 43,000 features. After an exploratory analysis, many of the sensors were found to be highly correlated. This could lead to a multicollinearity problem, which is when more than two independent variables are correlated. This issue can negatively affect model predictions on unseen data.
Artificial intelligence engineers from NineTwoThree were able to reduce the number of features from 43,680 to a more manageable and easier-to-analyze 136.
Modeling
Once all the sensor data is organized, a machine learning for manufacturing model is developed to predict degradation levels for each component of the hydraulic system. Understanding which models to use and which hypotheses to test can save a lot of time and training, thus achieving greater model accuracy more quickly.
For this project, four machine learning models were used to predict the state of each of the main components—cooler condition, valve condition, internal pump leakage, and hydraulic accumulator.
With accuracies greater than 99.0 percent, the models can now identify the inefficient performance of a component, and the manufacturer’s maintenance team receives an immediate notification of the problem. This alert provides data on exactly which component of the hydraulic system requires their attention and what the issue may be. This allows for intelligent, preventive maintenance to limit downtime, reduce maintenance costs, and extend the life of their equipment.
Whether a manufacturer has a high degree of data maturity or is just becoming aware of the benefits of predictive maintenance, employing machine learning is proving to be worth the investment.